TensorFlow 시작하기
TensorFlow를 사용하면 어떤 환경에서도 실행할 수 있는 ML 모델을 쉽게 만들 수 있습니다. 대화형 코드 샘플을 통해 직관적인 API를 사용하는 방법을 알아보세요.
import tensorflow as tf mnist = tf.keras.datasets.mnist (x_train, y_train),(x_test, y_test) = mnist.load_data() x_train, x_test = x_train / 255.0, x_test / 255.0 model = tf.keras.models.Sequential([ tf.keras.layers.Flatten(input_shape=(28, 28)), tf.keras.layers.Dense(128, activation='relu'), tf.keras.layers.Dropout(0.2), tf.keras.layers.Dense(10, activation='softmax') ]) model.compile(optimizer='adam', loss='sparse_categorical_crossentropy', metrics=['accuracy']) model.fit(x_train, y_train, epochs=5) model.evaluate(x_test, y_test)
ML을 사용해 실제 문제 해결하기
TensorFlow를 사용하여 어떻게 연구를 발전시키고 AI 기반 애플리케이션을 빌드하는지에 관한 예시를 살펴보세요.
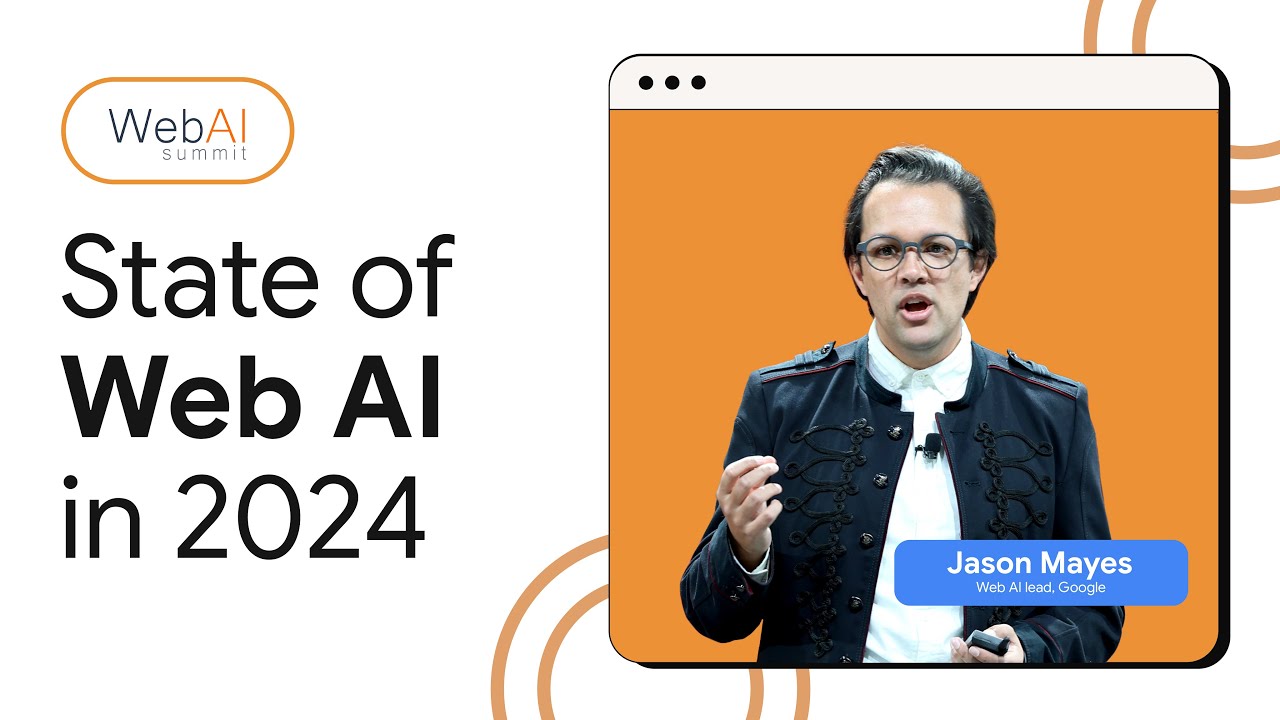
Explore the latest advancements in running models client-side with speakers from Chrome, MediaPipe, Intel, Hugging Face, Microsoft, LangChain, and more.
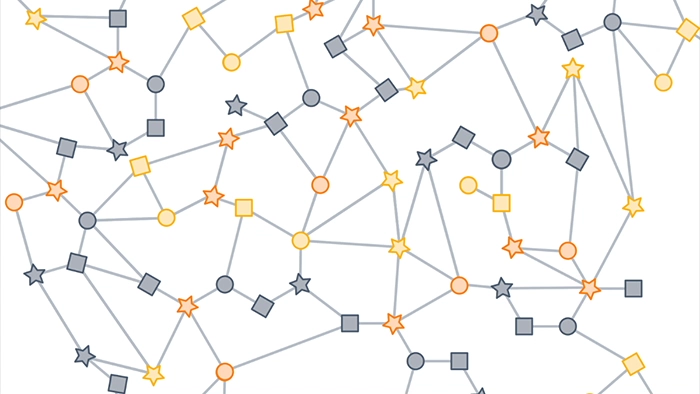
GNNs can process complex relationships between objects, making them a powerful technique for traffic forecasting, medical discovery, and more.
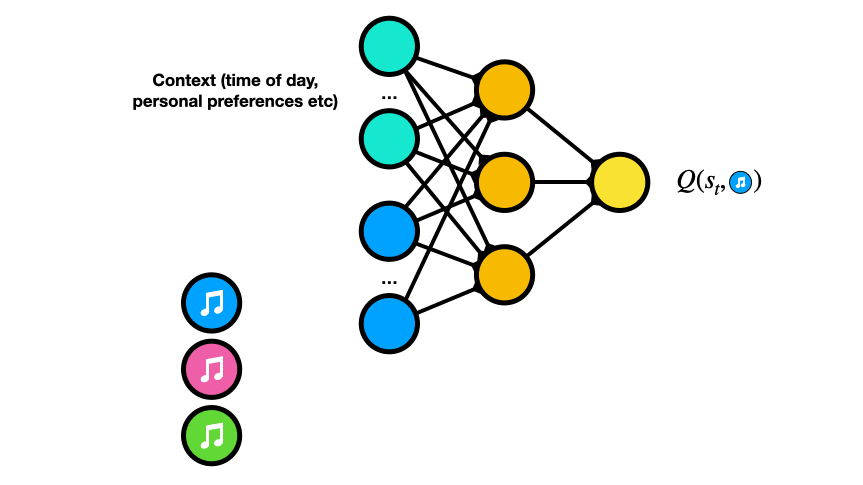
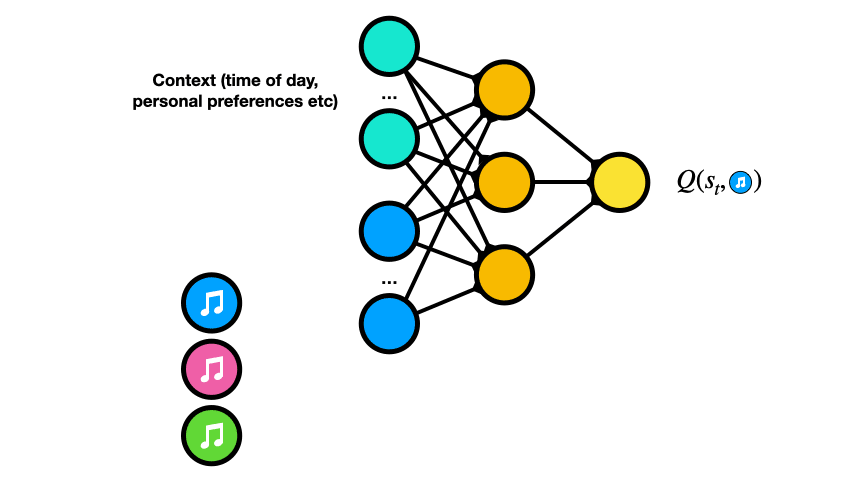
Spotify가 TensorFlow 생태계를 활용해 어떻게 확장 가능한 오프라인 시뮬레이터를 설계하고 RL 에이전트가 재생목록을 생성하도록 학습시키는지 알아보세요.
TensorFlow의 새로운 기능
TensorFlow팀 및 커뮤니티의 최신 공지사항을 읽어 보세요.
생태계 살펴보기
프로덕션 테스트를 거친 도구를 살펴보고 모델링, 배포, 기타 워크플로의 속도를 높여 보세요.
-
Library
TensorFlow.js
Train and run models directly in the browser using JavaScript or Node.js.
-
Library
LiteRT
Deploy ML on mobile and edge devices such as Android, iOS, Raspberry Pi, and Edge TPU.
-
API
tf.data
Preprocess data and create input pipelines for ML models.
-
Library
TFX
Create production ML pipelines and implement MLOps best practices.
-
API
tf.keras
Create ML models with TensorFlow's high-level API.
-
Resource
Kaggle Models
Find pre-trained models ready for fine-tuning and deployment.
-
Resource
TensorFlow Datasets
Browse the collection of standard datasets for initial training and validation.
-
Tool
TensorBoard
Visualize and track development of ML models.